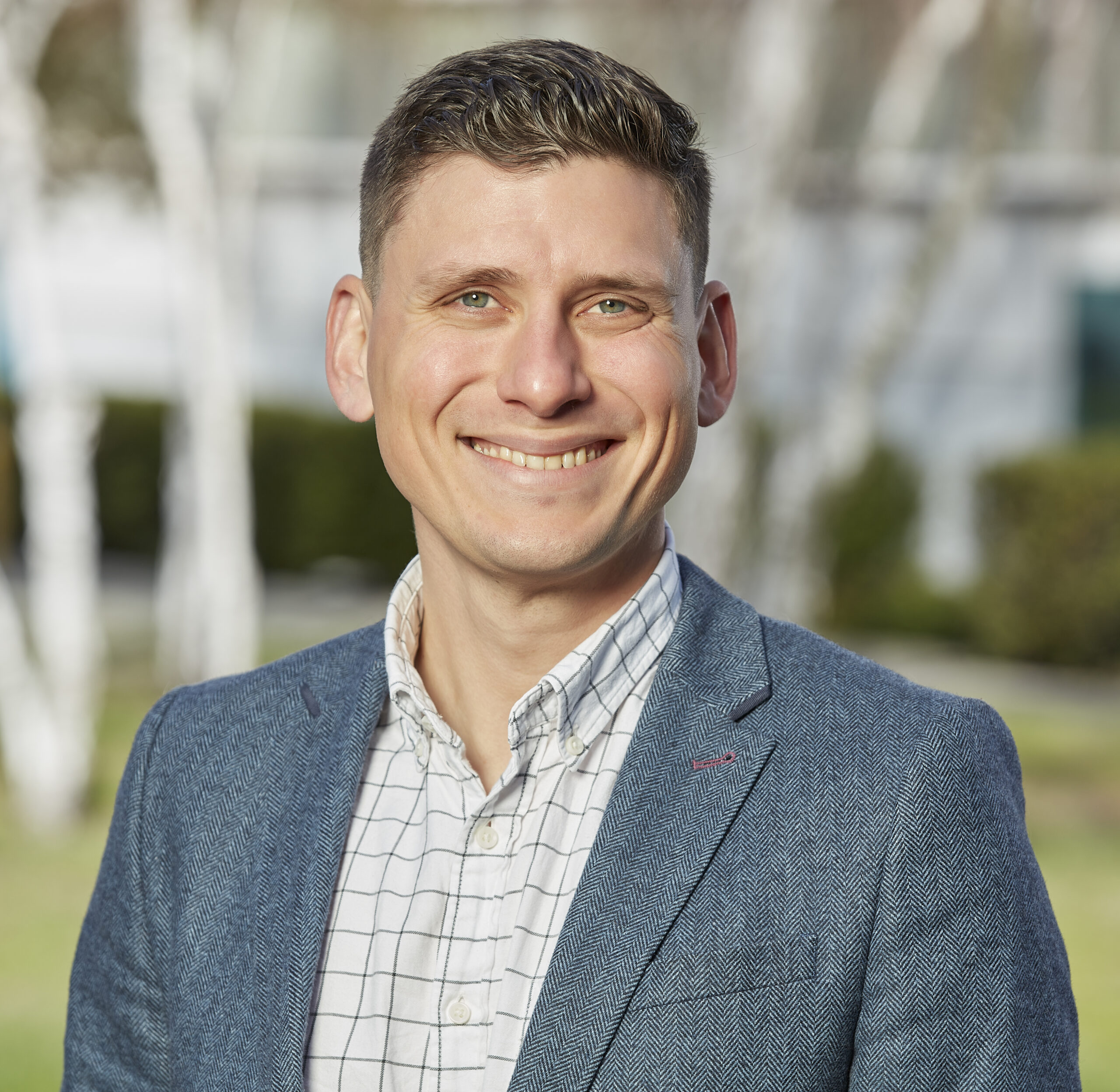
Abstract:
Engineering and research projects often involve optimizing a variable with respect to input parameters while respecting a constraint. For example, this might be optimizing the power production of a reactor by changing fuel parameters while maintaining a power peaking factor below a certain threshold. The design process can involve expensive modeling or physical experimentation, where the expense may be a combination of time, cost, manpower, or materials. Constrained Bayesian Optimization is a machine learning framework to optimize an engineered system while minimizing iterations of the resource intensive model or experiment. This seminar introduces the algorithm and shows its application to designing integral experiments for nuclear data validation, criticality safety, and advanced reactor neutronics mockups.
Bio:
Daniel Siefman became an assistant professor in the Nuclear Engineering Department in 2024. His research interests include critical and subcritical experiments and methods, nuclear data validation and adjustment, computational methods in radiation transport, neutron noise, reactor dosimetry, design optimization and safety analysis of nuclear reactors with machine learning, and nuclear power plant decommissioning. Daniel received a bachelor’s degree in Nuclear Engineering from the University of Florida in 2013, masters degrees in Nuclear Engineering from the École polytechnique fédérale de Lausanne (EPFL) and from ETH Zurich in 2015, and a PhD in Nuclear Engineering from EPFL in 2019. From 2019 to 2023, he was a staff scientist in the Nuclear Criticality Safety Division at Lawrence Livermore Laboratory supporting R&D efforts in integral experiments, nuclear data validation, radiation transport, neutron noise, and diagnostics for nuclear emergency response.